Mastering predictive lifetime value in the iOS 14.5+ era
Adjust's Katie Madding explores how to make accurate LTV predictions in a post-IDFA world
Making accurate predictions about the lifetime value (LTV) of users -- and finding more big spenders -- in the post-IDFA era often leaves marketers feeling like they need a crystal ball.
The reality is that for apps relying on in-app purchases for revenue, iOS 14.5+ has made data-driven decisions more difficult. With deterministic data in short supply, app marketers need to find new solutions to calculate predictive LTV (or pLTV) to secure the bottom line.
In terms of actual revenue, the post-IDFA world isn't actually impacting how much users spend in-app. In fact, in-app spending actually grew nearly 20% year-on-year from 2020 to 2021.
Any good iOS strategy in 2022 starts by focusing on getting user consent
However, the limited availability of deterministic attribution for opted-out users could make it harder for app publishers to know exactly how much revenue each campaign generated. Without a clear picture of the top-performing channels for user acquisition, marketers may struggle to pinpoint exactly where their biggest spenders are coming from -- and how to find more users just like them.
Luckily, there are some excellent strategic approaches that marketing teams can take to make the most of what data is available. Any good iOS strategy in 2022 starts by focusing on getting user consent. By maximizing the number of users that opt-in you can maintain a baseline of deterministic data to work from, for modelling or forecasting purposes. And by identifying key signals to optimize for, you can make Apple's SKAdNetwork system work for you.
SKAdNetwork for in-app purchases
SKAdNetwork (SKAN) was introduced by Apple in 2018, though it saw little adoption. The philosophy behind SKAN is that it provides a type of campaign measurement where data at the user level is not available. Now with iOS 14, Apple has made the SKAN framework -- with some expanded features -- the only way to access advertising performance data in cases where users choose to restrict developers' access to the IDFA via AppTrackingTransparency (ATT).
For many games, onboarding a user and explaining the value of IAPs can take longer than 24 hours
For apps that monetize via in-app purchases (IAPs), the short window into user behaviour -- just 24 hours -- can be a problem. For many games, onboarding a user and explaining the value of IAPs can take longer than 24 hours. If a user is willing to pay for extra lives, that urge might not happen until they reach the more challenging levels. That's difficult to track if you only have a 24-hour view post-install.
In practice, for now, at least, the granularity of data for most apps is going to be limited. Depending on the level of precision you require, you can track IAP behaviour with SKAdNetwork in two main ways.
Event-based measurement of in-app purchases
The first is using an event-based approach (also known as bit masking). SKAN provides space for 6-bits of downstream metrics, a number between 0 and 63 (or between 000000 and 111111 in binary), with an initial 24-hour timer. In this model, marketers assign each of the six bits to an event, and whether that corresponding bit is set to a 0 or a 1 tells you whether that event occurred.
Marketers will have the challenge of selecting the minimum amount of post-install information needed to calculate the LTV, ideally in less than 24-48 hours. The optimization signal will have fewer data points and a delay, forcing optimization strategies to change drastically, relying on a more contextual approach.
If you'd like to track six or fewer IAP events, then this technique can be used, where a bit is simply linked to each event, and you can track these conversions. If you're planning on optimizing toward key milestones, such as "complete tutorial", "complete level one" or "make a purchase," then a bit masking approach is perfect. However, if you want more detailed insights into ranges or scales of values, you can create buckets of "purchases" or some other metric.
Bucket-based approach to measuring in-app purchases
A bucket-based conversion value system allows you to define values that track how much users are spending in the first 24 hours. For gaming, e-commerce, delivery, or travel booking verticals, Average Order Value (AOV) is a commonly-used KPI that measures the amount spent by users in-app. If you're optimizing toward AOV, it's good to use buckets that encompass different total purchase values.
In a bucket-based approach, you might set up ranges between $1-$5, $6-$10, and so on, with a value returning in the conversion value postback that corresponds to each of these buckets.
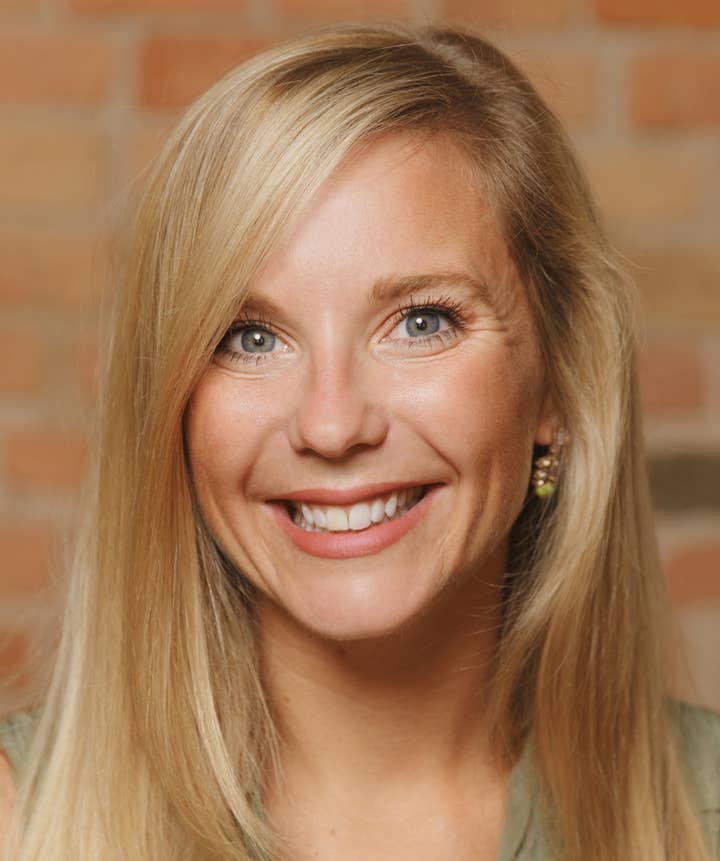
Predictive LTV modelling uses the behaviour of a user on their first day of using the app to predict revenue going forward in the medium term. Such predictive modelling works better when used for broader buckets or categories. You want to create wide definitions of possible success and filter users into these based on their behaviours. Using buckets to do broad strokes -- like dividing users into "whales" or "not-whales" -- is possible using users' initial behaviours.
By looking for day one spends that are outliers you can estimate how well your team is finding whales across different channels. If you create buckets that sort users into different broad categories on the first day, and then see how those categories are distributed across networks, you start to get a picture of where your highest-value users tend to come from.
For instance, if you create buckets for $1-10, $11-20, $21-30, you can be fairly confident that users in groups one and three have very different behaviours. A channel that is sending lots of users that are spending in the upper reaches of your value buckets is one that is sending a strong signal that it is able to reach high-value users. At the margins, it can be hard to pick apart how to read the information (is a user that spends $11 on day one really more valuable than one that spends $10?), but in broad strokes, you can see wider trends.
It is also possible to combine value-based signals with event flags, using some basic logic rules. For instance, you could set a value for a user that spends over $10 and buys a bundle of daily raid passes. An e-commerce app may find that users who buy consumables requiring them to return consistently are showing a stickier mentality than those who buy cosmetics, for example.
How AI can help improve predictions
Coming up with a strategy that works for your app is going to take some iteration, and as SKAdNetwork matures as a technology, best practice examples are going to change and advance. But the most important thing to focus on is learning what behaviours you want to encourage in your app -- and what actions high-value users are likely to take.
The most important thing to focus on is learning what behaviours you want to encourage in your app - and what actions high-value users are likely to take
AI, or machine learning, can analyse layers of trends that help to predict a user's future behaviour. AI uses historical data and patterns, learned from similar users, to predict the value of that user on, say, day 30, from data supplied on day 1. By working with large data sets fed into machine learning algorithms, AI can extrapolate and correlate to paint a picture of long-term outcomes for non-opted-in users.
By identifying these key points, and finding ways to maximize the touchpoints, you can create a good base with which to predict LTV going forward. The bottom line is that deterministic data remains the gold standard for making data-based decisions. However, with the right SKAdNetwork philosophy, you can ensure you are getting the best information possible from your opted-out users.
Katie Madding is chief product officer at Adjust, an analytics platform that helps marketers grow their mobile apps with solutions for measuring and optimizing campaigns, and protecting user data.
More GamesIndustry.biz Academy guides to Selling Games
Our guides to making money from video games cover various aspects of the publishing process, whether you're a young game developer about to start a new project or an industry veteran: